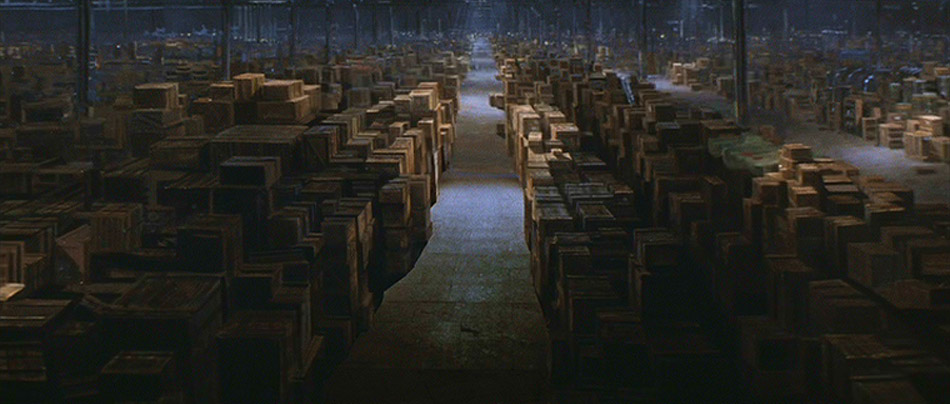 |
The Indiana Jones Warehouse |
The more stuff you own, the more time you spend managing it.
While this insight from my mother was brought to life when referring to clothing and home furnishings, it is hugely applicable to the world of data storage and analysis.
Whenever we start a data project with a client, we are always shocked by how much data they store that is simply not used. Not data that they generate, either. One of our clients
had an SQL database with 1,800 economic indicators stored for 210 countries going back several decades. After talking with analysts directly, we discovered there were three HUGE problems with this approach:
The first problem was that most of the data pulls included only
two core indicators: GDP and population. The second was that most of the data pulls were for
six unique countries (USA, Japan and the
BRICs). And the final problem was that various parts of the data were updated monthly, which meant that at least one member of the analyst team was responsible for refreshing this data twelve times a year,
taking him two to three full days to complete each update.
So, let's review:
The database in question had (1,800 indicators x 210 countries)
378,000 unique data series.
The most commonly used ones?
Only twelve.
The company was spending 200-300 man hours a year to update one data set - of which 99.99968% was almost never being used. The associated cost of updating this data doesn't even include direct maintenance, storage requirements or troubleshooting.
But why?
When asked why they were holding on to this much unused data, the client gave the response that all hoarders give:
"Because we might need it"
Obviously this is not a good reason to store gigabytes of superfluous data.
A successful database structure must be fluid enough that it can adapt to changing data requirements. Otherwise not only will you waste time storing and maintaining it, but your database will collapse under its own weight when changes arise.
Cure inventory waste
Here are four ways to prevent huge amounts of inventory waste in analytical databases.
1. Flexibility is everything. Flexibility allows you to store only the data you need; adding and subtracting data when your needs change. There are two ways to practice flexibility. First is the manner in which its structured and stored. SQL databases can be too rigid for some applications.
While it is good for some data, make sure you look at NoSQL options like MongoDB or CouchDB that can be better for storing price strips or economic indicators. Second,
inserting and retrieving data must be easy. Simply put, if it is hard to put new data in or pull data out then adjusting to new data requirements become nearly impossibles.
2. Think about the use cases first. This applies to reducing many different kinds of data waste, but database architects and analysts must come together on needs and requirements before engaging in a project.
Conversations between the two must be forward looking, thinking thoroughly about how the database will adapt to rapidly changing requirements.
3. Keep the data updated. This seems obvious, but data that is out of date helps no one. Keep it fresh through manual updating or an automated
ETL process, and it will be used regularly. Otherwise,
analysts will find different solutions (like going directly to the source) which will drive down the value of your database enormously.
4. Use analytics to track usage. IT must understand what data their analysts are using, how frequently and for what purpose. By using services like
Splunk,
Kibana or the analytics in
SQL Server, you can
detect which data is being used and by whom, keeping your database lean and useful.
Readers:
What did we miss? How do you and your team make sure that the data you maintain is being used effectively?